#DLD25: Bias, Power, and the Global AI Divide
- Romy Kraus
- Jan 18
- 3 min read
Humans in the AI Loop: Stanford's James Landay and Ina Fried Break It Down
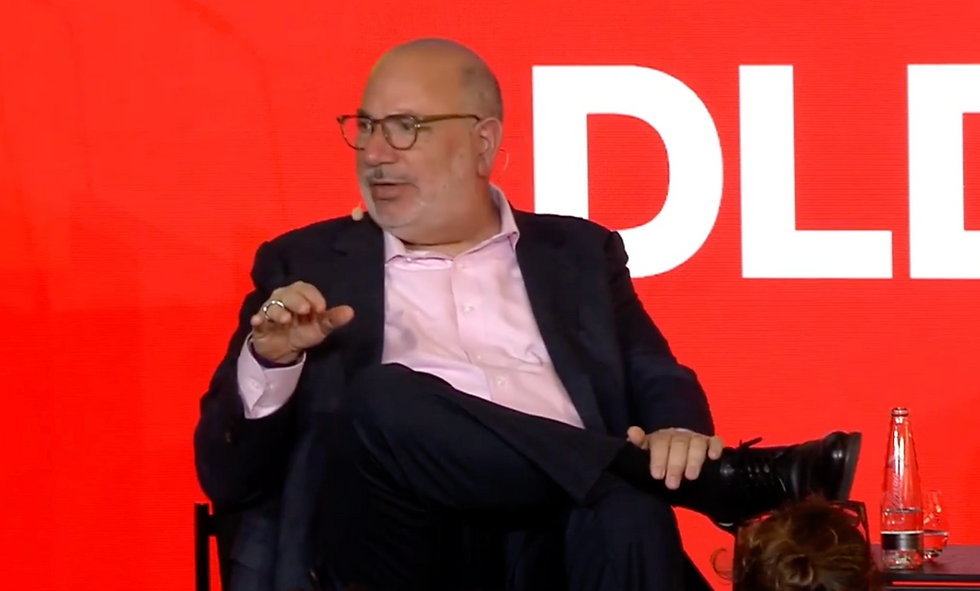
At DLD25, James Landay, a professor at Stanford and leader at the Stanford Institute for Human-Centered Artificial Intelligence (HAI), joined forces with Ina Fried, Chief Technology Correspondent at Axios, to unpack the challenges of making AI truly benefit humanity. Their conversation traversed the complexities of designing AI systems that consider everyone—not just the developers or direct users—and emphasized why embedding diverse perspectives in AI development is critical for its long-term societal impact.
From ethical pitfalls to practical steps for creating inclusive, equitable AI, the session was a roadmap for how academia, industry, and governments must collaborate to guide this transformative technology responsibly.
The Lowdown
Human-centered AI isn't just about user experience—it’s about societal outcomes.
AI perpetuates existing biases unless actively corrected, with most data skewing Western and English-based.
Academic institutions play a pivotal role in maintaining accountability and transparency in AI research.
Companies must diversify teams and embed ethical considerations early in development.
Democratizing AI requires focused resources to prevent wealthier, educated groups from monopolizing its benefits.
“AI for Good Isn’t Good Enough”
James Landay challenged the popular “AI for Good” narrative, arguing that good intentions don’t guarantee good outcomes. AI systems need to be built with broader societal impacts in mind, from healthcare to criminal justice. The concept of community-centered design broadens the lens to include indirect stakeholders, like patients' families in healthcare decisions or communities affected by criminal sentencing systems.
"You need to predict societal-level effects and mediate them early on." – James Landay
Bias in the System Runs Deep
AI systems mirror societal biases, as they're trained on existing, often Western-centric, data. Fixing bias isn’t just about removing it—it’s about deciding what’s acceptable. Landay noted the cultural disconnect when applying Western-trained AI in global contexts and the lack of transparency in the data driving major AI models.
"Most large models embed Western cultural values. How do we ensure they align with global needs?" – James Landay
Diversity: The Missing Ingredient
Lack of diversity in AI teams has led to notable failures, such as facial recognition systems that misidentify people of color. Landay stressed the need for diversity—not just in technical skills but also in lived experiences—to identify and fix problems early.
"Problems like biased facial recognition wouldn’t have happened if diverse voices were on those teams." – James Landay
Democratization Won’t Happen Automatically
Despite promises of AI democratizing healthcare and education, Landay argued this won’t happen without targeted strategies. Historically, new technologies first benefit wealthy, educated groups, and AI is no exception. Achieving equitable access requires investments from governments, nonprofits, and private companies to bridge the gap.
"AI will always benefit the rich first unless diffusion is intentional." – James Landay
Why Academia Still Matters
As private companies dominate AI innovation, Landay emphasized academia’s critical role in maintaining transparency and accountability. Universities and nonprofits need resources to build independent models and challenge corporate narratives, ensuring society understands how these systems work and their potential risks.
"Without academia, we won't understand why AI works the way it does—or how to improve it." – James Landay
Quickfire: Where Do We Go From Here?
What grade would you give AI’s current state?
A B-minus. Great progress, but glaring issues in inclusivity, bias, and societal impact.
What’s next for Stanford’s HAI?
Exploring neuroscience-inspired AI and common-sense reasoning.
Attracting top talent like new hire Yejin Choi, a leader in AI’s reasoning capabilities.
How do we ensure AI benefits everyone?
Embed diverse voices in product teams, invest in equitable access, and prioritize accountability through academic and nonprofit partnerships.
This session was more than just a discussion—it was a call to action. As Ina Fried and James Landay made clear, the future of AI isn’t just about making it smarter; it’s about making it fairer, more inclusive, and truly human-centered. The challenge is immense, but the roadmaps they offered point toward a future where AI works for everyone.